AI in Clinical Trials: Accelerating Research and Innovation
The world of clinical trials has always been a race against time—a race to bring safe, effective treatments to patients while managing the immense costs, complexities, and uncertainties inherent in medical research. For decades, the process remained linear and manual, often hindered by inefficiencies in patient recruitment, data collection, and trial management. However, artificial intelligence (AI) is now transforming this landscape, revolutionizing how clinical trials are designed, conducted, and analyzed.
AI is not just another tool in the researcher’s kit; it is reshaping the foundations of clinical research. By leveraging AI, pharmaceutical companies and researchers are finding new ways to reduce trial timelines, optimize patient selection, and generate real-time insights that enhance decision-making. This evolution is not just about speeding up drug development—it’s about unlocking innovation that improves patient outcomes and reshapes the future of medicine.
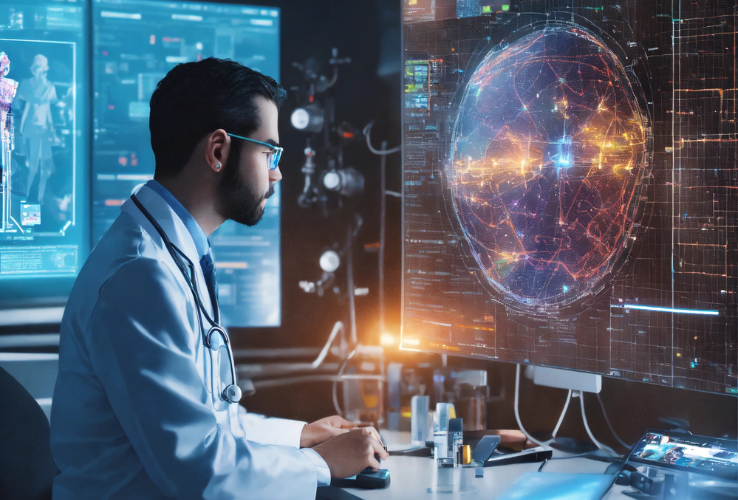
The Pain Points of Traditional Clinical Trials
Clinical trials have long been plagued by challenges that delay progress and inflate costs. Recruiting eligible patients, a critical first step, is one of the most significant bottlenecks. Studies show that 80% of trials fail to meet enrollment timelines, with over 30% of participants dropping out before completion. This not only slows research but also increases the financial burden on sponsors, with the average cost of developing a single drug now exceeding $1 billion.
Beyond recruitment, data collection and analysis often rely on labor-intensive processes prone to human error. Monitoring patient safety and treatment efficacy requires regular, manual updates that can lag behind real-world developments. This inefficiency extends trial durations and, in some cases, compromises the quality of the findings.
AI is addressing these challenges head-on, bringing a level of precision, efficiency, and scalability that was previously unattainable.
How AI is Revolutionizing Clinical Trials
1. Optimizing Patient Selection
One of the earliest and most critical stages of a clinical trial is identifying participants who meet the trial’s inclusion criteria. Traditional methods often involve manual reviews of patient records, leading to inefficiencies and missed opportunities. AI changes this by analyzing vast amounts of electronic health record (EHR) data, genomic information, and even social determinants of health to identify suitable candidates with unprecedented accuracy.
For instance, machine learning algorithms can identify patterns in patient data that predict eligibility, such as specific biomarkers or genetic profiles. This not only accelerates recruitment but also ensures that participants are more likely to benefit from the trial, improving retention rates.
2. Real-Time Data Monitoring
AI-powered systems enable real-time monitoring of patient data during trials, offering a level of visibility that was previously impossible. Wearable devices and sensors collect continuous streams of data on vital signs, activity levels, and medication adherence, feeding this information into AI platforms that detect anomalies or trends.
This real-time insight allows researchers to identify potential safety concerns, adjust protocols as needed, and ensure that the trial remains on track. For example, if a participant shows early signs of an adverse reaction, AI can flag this for immediate attention, potentially preventing escalation.
3. Accelerating Data Analysis
Traditionally, analyzing trial data is a labor-intensive process that can take months or even years. AI drastically reduces this timeline by automating data aggregation, cleansing, and interpretation. Advanced algorithms can identify correlations and trends that might elude human analysts, uncovering insights that lead to faster and more informed decision-making.
In oncology trials, for example, AI has been used to analyze imaging data to track tumor progression. These systems can compare thousands of scans in a fraction of the time it would take a radiologist, providing accurate assessments of treatment efficacy.
Case Studies: AI in Action
AI-Powered Recruitment at Pfizer
Pfizer’s use of AI in patient recruitment for a COVID-19 vaccine trial exemplifies the technology’s impact. By analyzing electronic medical records and demographic data, AI systems identified eligible participants more quickly than traditional methods. This efficiency played a crucial role in accelerating the trial and delivering a vaccine in record time.
Real-Time Monitoring with Wearables
In a recent diabetes trial, wearable glucose monitors fed continuous data into AI platforms, providing researchers with minute-by-minute updates on blood sugar levels. This allowed for real-time adjustments to treatment protocols, improving patient safety and optimizing trial outcomes.
Accelerating Oncology Insights
A pharmaceutical company developing a targeted cancer therapy leveraged AI to analyze genomic data from trial participants. The system identified specific genetic mutations correlated with positive treatment responses, enabling the company to refine its inclusion criteria for subsequent trial phases.
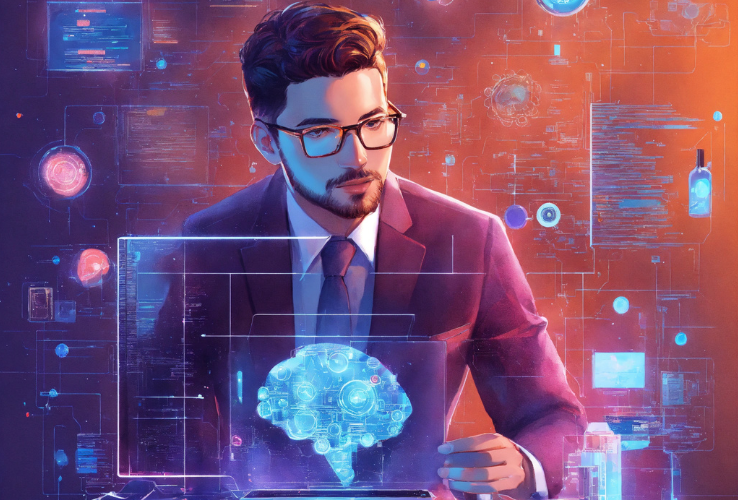
Challenges and Considerations in AI Adoption
While AI offers significant advantages, its integration into clinical trials is not without challenges:
Data Quality and Integration
AI systems rely on high-quality data, yet clinical trial data often comes from diverse sources with varying formats and standards. Ensuring data consistency and interoperability is critical for accurate analysis.Regulatory Compliance
The use of AI in clinical trials must align with stringent regulatory standards. Agencies like the FDA and EMA are still developing frameworks for validating AI-driven methodologies, which can slow adoption.Bias in Algorithms
AI models are only as unbiased as the data they are trained on. If training data lacks diversity or contains inherent biases, the resulting algorithms may produce skewed outcomes, potentially affecting trial results.Cost of Implementation
While AI promises cost savings in the long term, the initial investment in technology, infrastructure, and expertise can be substantial, particularly for smaller organizations.
The Future of AI in Clinical Research
The adoption of AI in clinical trials is expected to expand rapidly, driven by advancements in technology and increasing demand for faster, more efficient drug development. Key trends include:
Integration with Precision Medicine
AI will play a pivotal role in integrating genomic and real-world data into clinical trials, enabling highly targeted therapies and personalized treatment approaches.Decentralized Trials
AI will facilitate decentralized clinical trials, where participants can engage from their homes rather than visiting centralized locations. This will enhance patient accessibility and diversity while reducing trial costs.Predictive Modeling for Trial Design
AI algorithms will soon be able to predict the success of trial designs before they are implemented, allowing researchers to refine protocols and improve outcomes.