Edge Computing: Transforming Cloud Infrastructure for the IoT Era
The rapid rise of the Internet of Things (IoT) has reshaped how businesses and consumers interact with technology. From smart home devices and connected vehicles to industrial sensors and healthcare monitors, IoT is generating unprecedented volumes of data. However, the traditional cloud-centric model, while robust, is being tested by the demands of these latency-sensitive and data-heavy applications. Enter edge computing—a paradigm shift that processes data closer to its source, reducing latency and enhancing efficiency.
This transformation isn’t just about technology; it’s about enabling innovation. Imagine a manufacturing plant that uses hundreds of IoT sensors to monitor equipment performance in real time. Waiting for data to travel to a distant cloud server and back isn’t an option when split-second decisions are needed to prevent downtime. With edge computing, the data is processed locally, ensuring immediate action. This example illustrates how edge computing complements cloud infrastructure, delivering speed and agility for the IoT era.
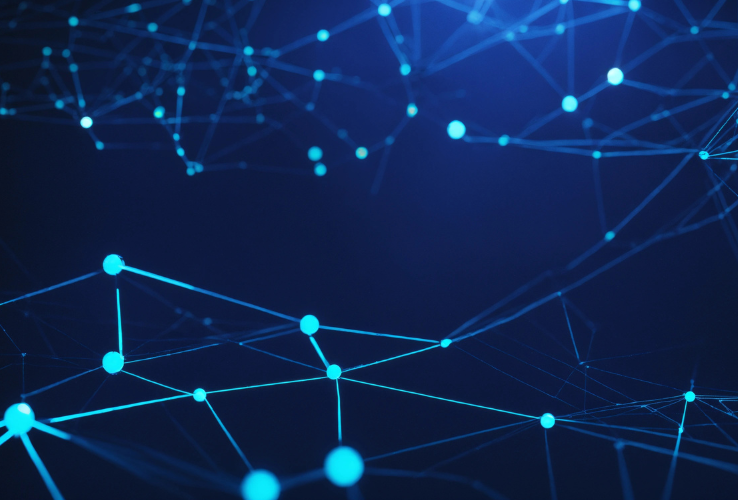
The Evolution of Edge Computing
Edge computing emerged as a solution to the limitations of centralized cloud systems. While cloud computing excels at providing scalable storage and computational power, its reliance on distant data centers introduces latency and bandwidth challenges for applications requiring real-time processing.
Edge computing shifts the computational workload closer to where data is generated—the “edge” of the network. This could be a smart device, a local server, or a gateway. By processing data locally, edge computing reduces the need for constant communication with the cloud, enhancing speed, reliability, and security.
For businesses, this evolution represents a new era of possibilities. It’s not about replacing the cloud but extending its capabilities, creating a hybrid ecosystem where edge and cloud work in harmony.
Applications of Edge Computing in IoT
1. Smart Cities: Enhancing Urban Efficiency
Edge computing is the backbone of smart city initiatives, where IoT devices like traffic cameras, environmental sensors, and public safety systems generate vast amounts of data. Processing this data at the edge allows cities to respond in real time to changing conditions, such as rerouting traffic to avoid congestion or deploying emergency services more efficiently.
For instance, a city using edge computing can analyze traffic patterns locally and adjust traffic lights dynamically, reducing delays and emissions. By offloading real-time decisions to edge nodes, smart cities can operate more efficiently without overburdening centralized cloud systems.
2. Industrial IoT: Powering Predictive Maintenance
In manufacturing, edge computing enables predictive maintenance by analyzing sensor data from equipment in real time. By detecting anomalies and predicting failures before they occur, edge computing reduces downtime and increases operational efficiency.
Consider an automotive assembly line where IoT sensors monitor machinery. An edge device processes data locally, identifying patterns that indicate potential equipment failure. Maintenance teams receive instant alerts, allowing them to address issues proactively, avoiding costly disruptions.
3. Healthcare: Supporting Critical Applications
Edge computing is revolutionizing healthcare by enabling real-time analysis of patient data from IoT devices like wearable monitors and medical imaging systems. This capability is especially critical in latency-sensitive scenarios, such as remote surgeries or emergency care.
For example, during a telemedicine consultation, an edge device processes vital signs locally, ensuring doctors receive real-time data without delays. In rural or remote areas with limited connectivity, edge computing ensures that essential healthcare services remain uninterrupted.
Addressing Latency and Bandwidth Challenges
Latency—the delay between data generation and processing—is a critical issue for IoT applications. In scenarios like autonomous vehicles or industrial automation, even a few milliseconds can make a difference. Edge computing addresses this challenge by processing data closer to its source, minimizing the time required for data to travel back and forth to the cloud.
Bandwidth is another constraint as IoT devices proliferate. Continuously transmitting massive amounts of data to the cloud can overwhelm networks and incur high costs. By filtering and processing data locally, edge computing reduces the volume of data sent to the cloud, optimizing bandwidth usage and lowering operational expenses.
For instance, a retail store using IoT cameras for inventory tracking processes video streams locally to identify stock shortages. Only relevant insights, such as low inventory alerts, are sent to the cloud, saving bandwidth and storage.
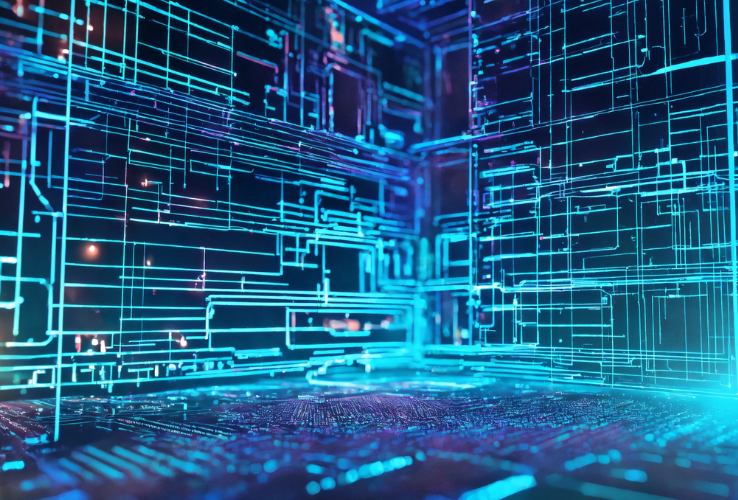
Challenges and Considerations in Edge Computing
While the benefits of edge computing are clear, its implementation is not without challenges:
- Security Concerns: Processing data at multiple edge nodes increases the attack surface, requiring robust security measures to protect sensitive information.
- Scalability: Managing a distributed network of edge devices can be complex, particularly as the number of IoT devices continues to grow.
- Interoperability: Ensuring that edge devices from different vendors work seamlessly together is essential for creating a cohesive system.
Addressing these challenges requires a combination of advanced tools, standardized protocols, and strategic planning. Organizations must adopt a holistic approach, integrating edge computing into their broader IT infrastructure while prioritizing security and scalability.
The Role of Cloud in an Edge-Driven World
While edge computing excels at local processing, the cloud remains indispensable for tasks like long-term data storage, large-scale analytics, and machine learning model training. Together, edge and cloud create a hybrid model that leverages the strengths of both.
For example, a logistics company using edge devices to track vehicle locations processes real-time data locally to optimize routes. Meanwhile, historical data from all vehicles is aggregated in the cloud, where advanced analytics identify trends and improve future operations.
This symbiotic relationship ensures that businesses can scale their operations, maintain agility, and derive actionable insights from their data.
The Future of Edge Computing
As IoT adoption accelerates, the role of edge computing will continue to expand. Emerging trends include:
- AI at the Edge: The integration of AI capabilities into edge devices enables real-time decision-making without relying on the cloud. This is particularly valuable in applications like autonomous vehicles and personalized healthcare.
- 5G Integration: The rollout of 5G networks enhances the capabilities of edge computing by providing ultra-low latency and high-speed connectivity, enabling more complex and data-intensive applications.
- Decentralized Architectures: Advances in blockchain and distributed computing are paving the way for decentralized edge networks, offering new levels of security and efficiency.
Conclusion: Empowering the IoT Era
Edge computing is not just a technological evolution—it’s a fundamental shift in how businesses approach data processing and decision-making. By bringing computation closer to the source, edge computing addresses the unique challenges of IoT, from latency and bandwidth constraints to real-time responsiveness.
For organizations navigating the complexities of the IoT era, adopting edge computing is not merely an option—it’s a strategic imperative. By combining the agility of edge with the scalability of cloud, businesses can unlock new possibilities, from smarter cities and efficient industries to transformative healthcare solutions.
As we move forward, the fusion of edge computing and IoT will continue to drive innovation, shaping a future where technology doesn’t just connect us—it empowers us. The edge is not just where data is processed; it’s where the future begins.