Building a Robust Data Strategy for AI Implementation
The journey to successful AI implementation is as much about data as it is about algorithms and models. While AI often takes the spotlight for its transformative potential, the unsung hero behind every impactful AI project is a well-crafted data strategy. Without a strong foundation of high-quality, well-governed, and accessible data, even the most sophisticated AI initiatives are destined to falter.
Organizations venturing into AI often find themselves grappling with fragmented data systems, inconsistent quality, and insufficient governance. These challenges can derail projects, waste resources, and erode trust in AI outputs. To harness the full potential of AI, businesses must start with a robust data strategy—one that not only addresses technical requirements but also aligns with organizational goals and compliance needs.
This is the story of why and how data strategy serves as the cornerstone of AI success.
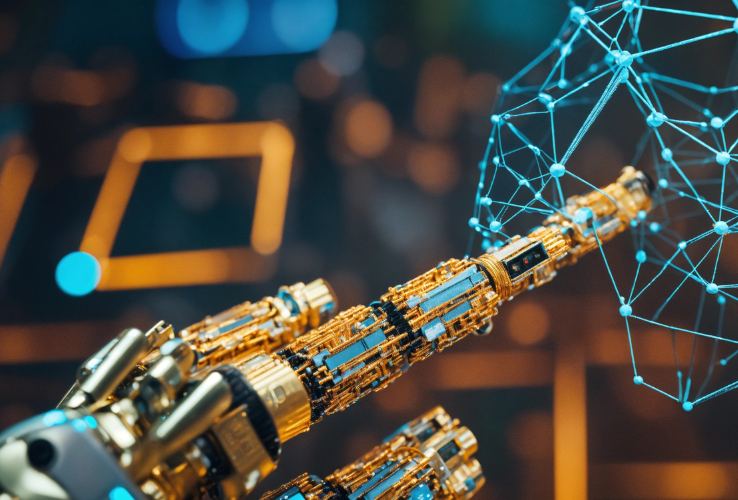
The Role of Data in AI: More Than Just Input
At its core, AI thrives on data. Machine learning models learn from historical data to make predictions, while natural language processing systems rely on vast datasets to understand context. The quality and relevance of data directly impact the accuracy, reliability, and fairness of AI systems.
Imagine a healthcare provider implementing AI to predict patient outcomes. If the data fed into the system is incomplete, outdated, or biased, the resulting predictions could be inaccurate, potentially jeopardizing patient safety. Conversely, a well-defined data strategy ensures that the AI system is trained on clean, representative data, leading to actionable insights that improve care delivery.
A robust data strategy is not merely about collecting vast amounts of data—it’s about collecting the right data, ensuring it’s managed effectively, and preparing it for analysis. This requires a concerted focus on governance, quality, and infrastructure.
Key Pillars of a Robust Data Strategy
1. Data Governance: Establishing Trust and Accountability
Data governance is the foundation of a successful data strategy. It encompasses the policies, processes, and frameworks that ensure data is accurate, secure, and compliant with regulations. In the context of AI, governance plays a critical role in ensuring that data is ethically sourced, free from bias, and used responsibly.
For example, a financial services firm leveraging AI for credit risk assessment must implement governance policies to avoid discriminatory practices. This involves ensuring that data inputs do not disproportionately exclude certain demographic groups and that decision-making processes are transparent and auditable.
Effective data governance also fosters trust among stakeholders. When teams are confident in the quality and security of their data, they are more likely to embrace AI solutions, accelerating adoption across the organization.
2. Data Quality: Feeding AI with the Right Ingredients
The adage “garbage in, garbage out” rings especially true for AI. Poor-quality data—whether due to inaccuracies, inconsistencies, or duplication—can lead to unreliable outcomes, undermining the credibility of AI systems.
Take the example of a retail company implementing AI for demand forecasting. If sales data is riddled with errors, such as incorrect product categorizations or missing entries, the AI system may fail to predict trends accurately, resulting in overstock or stockouts. A data quality strategy involves rigorous validation, cleansing, and enrichment processes to ensure that data is complete, accurate, and fit for purpose.
Beyond quality, the diversity of data also matters. AI systems trained on narrow datasets may struggle to generalize to real-world scenarios, limiting their effectiveness. Ensuring a representative dataset helps mitigate this risk, enabling AI to perform consistently across varied contexts.
3. Data Infrastructure: Building for Scalability and Speed
AI requires an infrastructure capable of handling large volumes of data and supporting complex computations. This includes scalable storage solutions, high-performance processing capabilities, and efficient data pipelines that ensure seamless data flow across the organization.
Cloud platforms have emerged as a critical enabler of AI-friendly data infrastructure. They provide the flexibility to scale storage and processing resources on demand while enabling access to advanced tools for data integration and analytics. For instance, a logistics company using AI to optimize delivery routes can leverage cloud-based infrastructure to process real-time traffic data and generate recommendations in seconds.
Additionally, modern data architectures like data lakes and data warehouses play a pivotal role in organizing and storing data. While data lakes offer flexibility for storing raw data, data warehouses are optimized for structured queries, making them ideal for AI projects that require clean, curated datasets.
Real-World Examples: Data Strategy in Action
1. Healthcare: Improving Patient Outcomes
A global healthcare organization embarked on an AI project to predict patient readmissions and improve care quality. The initiative began with a comprehensive data strategy, focusing on integrating patient records from multiple sources, standardizing data formats, and ensuring compliance with privacy regulations like HIPAA. By prioritizing data governance and quality, the organization was able to develop an AI model that accurately identified at-risk patients, enabling proactive interventions and reducing readmissions by 15%.
2. Retail: Personalizing the Customer Experience
A leading e-commerce platform aimed to enhance customer engagement using AI-driven recommendations. The company’s data strategy emphasized collecting and analyzing user behavior data, such as browsing history and purchase patterns, while ensuring transparency in data usage to build customer trust. With a robust infrastructure in place, the platform delivered highly personalized product recommendations, resulting in a 20% increase in sales.
3. Financial Services: Mitigating Risk
A multinational bank leveraged AI to detect fraudulent transactions in real time. The success of the project hinged on a robust data strategy that included high-quality transactional data, strict governance frameworks, and a scalable cloud infrastructure. By enabling real-time analysis and decision-making, the bank reduced fraud losses by 30% and improved customer confidence.
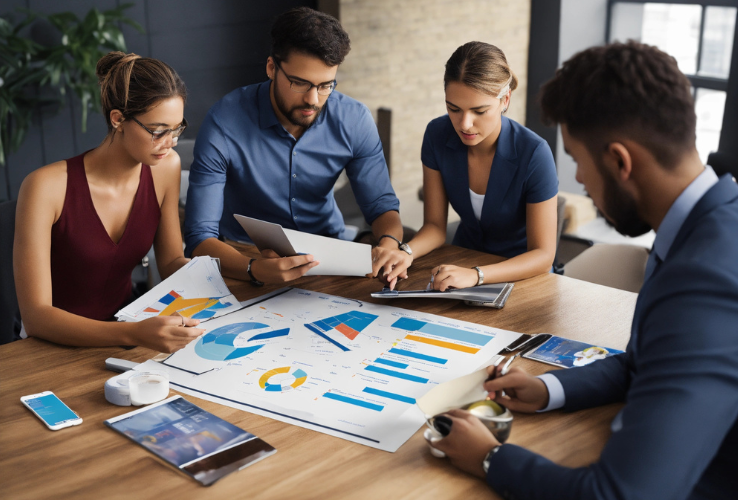
Why a Data Strategy Matters for AI Implementation
A well-defined data strategy is more than a technical necessity—it’s a competitive advantage. It ensures that AI systems are built on a foundation of trust, reliability, and efficiency, enabling organizations to maximize the value of their investments. Key benefits include:
- Reduced Risk: By addressing issues like data bias, security, and compliance upfront, organizations minimize the risks associated with AI deployment.
- Improved Efficiency: Clean, well-structured data reduces the time and effort required for data preparation, accelerating AI development cycles.
- Scalability: A strong data infrastructure supports the seamless expansion of AI initiatives, enabling organizations to scale their capabilities as needs evolve.
Conclusion: Building for Success
AI holds immense potential to transform industries, but its success depends on the strength of the data strategy that underpins it. From governance and quality to infrastructure and scalability, each component plays a critical role in ensuring that AI systems deliver accurate, actionable insights.
For organizations embarking on AI initiatives, investing in a robust data strategy is not just prudent—it’s essential. By laying a solid foundation, businesses can unlock the full potential of AI, driving innovation, efficiency, and growth in an increasingly data-driven world. With a strong data strategy in place, AI becomes not just a tool for today but a catalyst for a smarter, more connected future.