AI in Risk Management: Predictive Analytics for Financial Stability
In the intricate and fast-moving world of finance, risk management has always been a cornerstone of stability and success. As financial institutions face a growing array of risks—ranging from credit defaults to market volatility and cyber threats—the traditional tools of risk management are proving inadequate. Enter artificial intelligence (AI), a transformative force that is redefining how banks identify, evaluate, and mitigate risks. With predictive analytics at its core, AI is equipping financial leaders with insights and strategies that were once unimaginable.
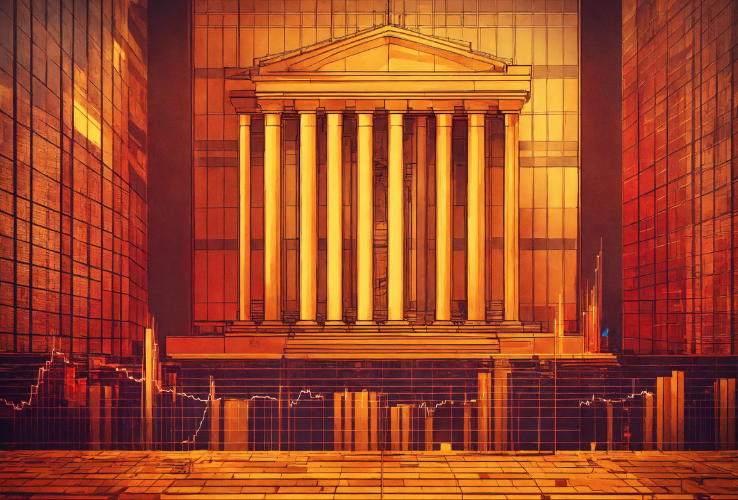
The Role of Predictive Analytics in Risk Management
Predictive analytics leverages historical and real-time data to forecast potential risks and opportunities. By applying machine learning algorithms and statistical models, financial institutions can uncover hidden patterns, anticipate future events, and make data-driven decisions with unprecedented accuracy.
In the realm of risk management, predictive analytics offers several critical advantages:
- Proactive Risk Mitigation: Instead of reacting to risks after they materialize, banks can now anticipate and address them before they escalate.
- Enhanced Decision-Making: Predictive models provide decision-makers with actionable insights, enabling them to allocate resources more effectively and mitigate financial exposure.
- Efficiency Gains: AI-powered tools automate labor-intensive processes, freeing up human resources for strategic tasks.
Key Applications of AI in Risk Management
1. Credit Risk Assessment
For example, machine learning algorithms can identify subtle patterns in borrower behavior that may indicate future defaults, enabling banks to adjust credit limits or interest rates proactively.
2. Fraud Detection and Prevention
AI excels at detecting anomalies that might signal fraudulent activity. Unlike rule-based systems, AI models adapt and evolve as new fraud patterns emerge. This capability is particularly valuable in identifying sophisticated schemes, such as synthetic identity fraud or phishing attacks.
For instance, real-time monitoring tools powered by AI can flag unusual transactions—such as large withdrawals or purchases in different locations—prompting immediate action to prevent losses.
3. Market Risk Management
Volatile markets pose significant risks to financial institutions, but AI’s ability to process and analyze high-frequency data provides a critical advantage. Predictive models can simulate market scenarios, assess portfolio vulnerabilities, and recommend hedging strategies to minimize exposure.
Algorithmic trading platforms also use AI to predict price movements and execute trades, balancing risk and reward in real time.
4. Operational Risk Monitoring
Operational risks, such as system failures or regulatory non-compliance, can have far-reaching consequences. AI-powered tools monitor internal processes, flagging irregularities and ensuring adherence to compliance standards. Natural language processing (NLP) tools, for example, can analyze vast amounts of regulatory text to ensure that a bank’s policies align with evolving legal requirements.
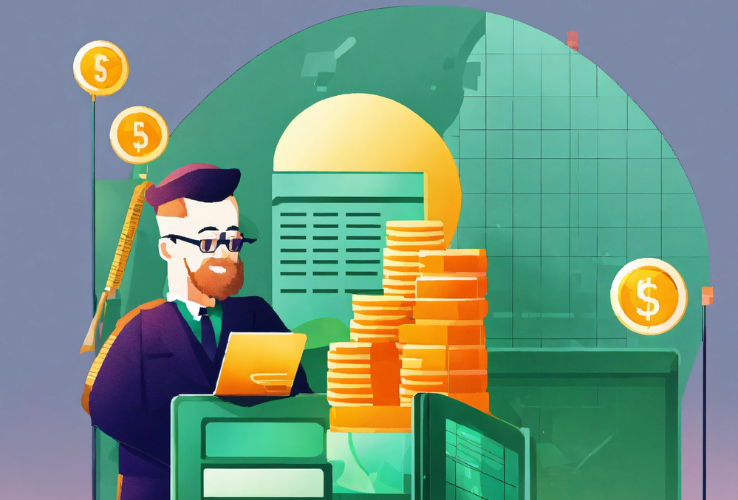
Tools and Technologies Driving AI Adoption in Risk Management
Machine Learning Algorithms
Machine learning models are the backbone of predictive analytics, enabling banks to analyze complex datasets and make accurate predictions. Techniques like supervised and unsupervised learning allow for both the identification of known risks and the discovery of new ones.
Natural Language Processing (NLP)
NLP tools process unstructured data—such as news articles, social media posts, or regulatory updates—to extract valuable insights. For instance, sentiment analysis can gauge public reactions to market events, informing risk management strategies.
Robotic Process Automation (RPA)
RPA tools enhance operational efficiency by automating routine tasks like data entry, report generation, and compliance checks. When integrated with AI, RPA becomes even more powerful, handling complex workflows with minimal human intervention.
Big Data Analytics
AI thrives on data, and big data platforms provide the infrastructure needed to process and analyze vast datasets. Cloud-based solutions like AWS or Google BigQuery allow banks to scale their AI initiatives seamlessly.
The Impact on Decision-Making
The adoption of AI in risk management has transformed decision-making in several ways:
- Data-Driven Insights: AI enables leaders to move beyond intuition, relying instead on evidence-based insights for more accurate decisions.
- Real-Time Responsiveness: Predictive analytics allows banks to respond to risks as they arise, minimizing potential losses and safeguarding financial stability.
- Strategic Resource Allocation: By identifying high-risk areas, AI helps organizations focus their resources where they are needed most, improving efficiency and effectiveness.
Challenges and Considerations
While AI offers tremendous potential, its implementation is not without challenges:
- Data Quality: Predictive models are only as good as the data they analyze. Ensuring data accuracy, completeness, and relevance is critical for reliable outcomes.
- Regulatory Concerns: The use of AI in financial services raises questions about transparency, accountability, and compliance with evolving regulations.
- Bias and Fairness: Machine learning models may inadvertently perpetuate biases present in historical data, leading to unfair or discriminatory outcomes.
- Integration Costs: Adopting AI requires significant investment in technology, infrastructure, and talent, which may be prohibitive for smaller institutions.
The Future of AI in Risk Management
As AI technologies continue to evolve, their role in risk management will only expand. Future advancements may include:
- Explainable AI (XAI): Tools that provide transparency into how AI models make decisions, addressing regulatory and ethical concerns.
- Quantum Computing: The integration of quantum computing with AI could exponentially enhance the speed and accuracy of predictive models.
- Continuous Learning Systems: AI models that adapt in real-time to new data and changing conditions, ensuring they remain effective in dynamic environments.
A Strategic Imperative for Financial Stability
AI in risk management is not just a technological upgrade; it’s a strategic imperative for financial institutions seeking to navigate an increasingly complex and volatile landscape. By leveraging predictive analytics, banks can anticipate risks, protect their assets, and maintain financial stability in an uncertain world.
For leaders in the financial sector, the question is no longer whether to adopt AI but how to do so effectively. With the right tools, strategies, and governance, AI can transform risk management from a reactive necessity into a proactive advantage—paving the way for a more secure and resilient financial ecosystem.