Supply Chain Resilience: Overcoming Disruptions with Predictive Analytics
In the interconnected world of manufacturing and logistics, supply chain disruptions can ripple across industries, affecting production schedules, delivery commitments, and ultimately, customer satisfaction. Recent global events have underscored the fragility of traditional supply chain systems, pushing enterprises to rethink their strategies. At the heart of this evolution lies predictive analytics—a powerful tool that transforms uncertainty into actionable insights.
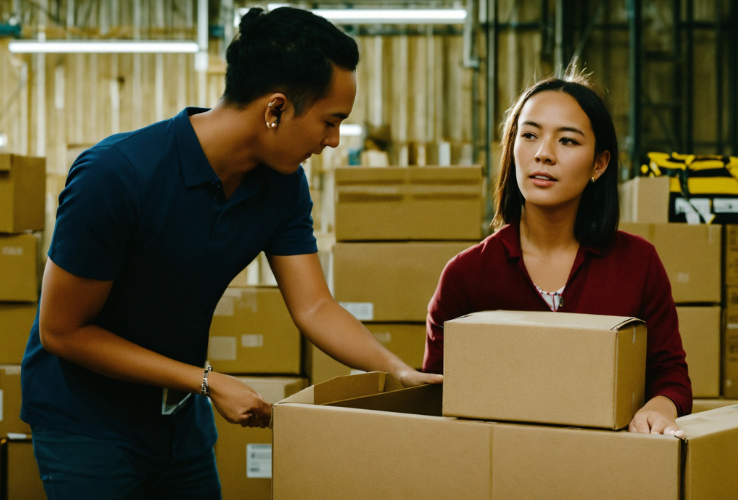
The Complexities of Modern Supply Chains
Supply chains today are intricate ecosystems. A single product may pass through multiple regions, suppliers, and modes of transport before reaching its final destination. This complexity introduces vulnerabilities to various risks, such as geopolitical tensions, natural disasters, labour strikes, and unexpected demand fluctuations. Traditional methods of risk management, often reactive in nature, struggle to keep pace with these dynamic challenges.
Predictive analytics offers a proactive approach. By leveraging data from across the supply chain—including historical performance, weather patterns, market trends, and real-time operational metrics—businesses can anticipate potential disruptions and respond before they escalate into crises.
The "Why" Behind Predictive Analytics
Why are companies increasingly turning to predictive analytics for supply chain resilience? The answer lies in its ability to provide foresight and precision. Unlike descriptive analytics, which focuses on past events, predictive analytics delves into future possibilities. Here’s why this shift is pivotal:
Risk Identification: Predictive models can highlight vulnerabilities before they materialize. For instance, a manufacturer sourcing materials from a region prone to flooding can prepare alternate supply routes during high-risk seasons.
Optimised Decision-Making: Advanced algorithms process vast amounts of data to recommend the best course of action. Whether it’s rerouting shipments or reallocating inventory, decisions are backed by data-driven insights.
Cost Efficiency: By preempting disruptions, businesses can avoid expensive last-minute fixes, such as expedited shipping or emergency supplier engagements.
How Predictive Analytics Works in Supply Chain Management
The implementation of predictive analytics in supply chain operations is not merely about deploying technology; it’s about integrating it into the decision-making fabric of the organisation. Here’s how global enterprises are achieving this:
Data Collection and Integration: Enterprises aggregate data from multiple sources, including IoT devices, ERP systems, weather forecasting platforms, and social media. This comprehensive dataset forms the foundation for predictive models.
Advanced Algorithms: Machine learning models analyse patterns within the data, identifying correlations and anomalies that may indicate potential risks.
Scenario Simulation: Predictive tools allow businesses to simulate various scenarios, such as a supplier shutdown or a surge in demand. These simulations help assess the impact of potential disruptions and evaluate contingency plans.
Real-Time Monitoring: Dynamic dashboards and alerts ensure that decision-makers are informed as situations evolve, enabling swift and informed responses.
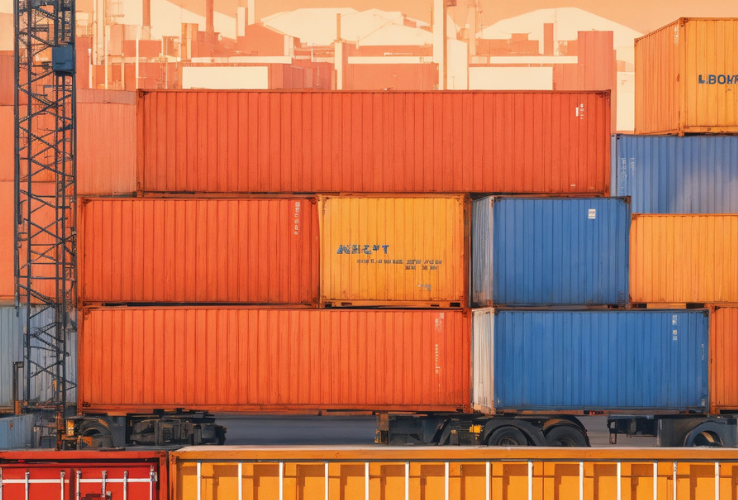
Case Studies: Lessons from Industry Leaders
Several global enterprises have embraced predictive analytics to navigate supply chain challenges successfully:
Case Study 1: Automotive Industry
A leading automaker faced recurring delays in semiconductor supplies due to demand-supply imbalances. By deploying predictive analytics, the company identified potential bottlenecks months in advance and diversified its supplier base. This strategic move reduced lead times by 30% and safeguarded production schedules.
Case Study 2: Retail and E-Commerce
An e-commerce giant leveraged predictive analytics to manage demand spikes during peak shopping seasons. By analysing historical sales data and weather patterns, it optimised inventory placement across warehouses, reducing delivery delays and cutting operational costs by 15%.
Challenges and Considerations
While the benefits of predictive analytics are compelling, its adoption is not without challenges. Data silos, inconsistent data quality, and the need for skilled talent to manage advanced analytics tools can hinder implementation. Businesses must also address concerns around data privacy and ensure compliance with evolving regulations.
The Path Forward
For manufacturers and logistics providers aiming to build resilient supply chains, predictive analytics is no longer a luxury; it’s a necessity. The journey begins with a commitment to data-driven transformation and a willingness to invest in the right technologies and expertise.
As the supply chain landscape grows increasingly volatile, those who embrace predictive analytics will not only mitigate risks but also seize opportunities. By transforming data into foresight, these organisations can navigate uncertainty with confidence and ensure smooth, uninterrupted operations—even in the face of disruption.